"Be cautious of the fact that aerial and drone imagery are generally not well calibrated. Any outputs of quantitative analyses (e.g. vegetation indices) of such imagery should be interpreted with great care, especially when comparing images that were acquired at different times of the day or year. "
DigitalGlobe’s WorldView-3/4 satellites currently provide the highest resolution (30 cm) imagery available for public consumption. It is unlikely that higher resolution satellite images will become available any time soon as DigitalGlobe had quite a hard time convincing the US federal government to permit 30cm imagery for non-military use.
In contrast, the spatial resolution of aerial (including drone or unmanned aerial vehicle) imagery is nearly limitless as it essentially depends on the altitude from which the imagery is acquired. The higher resolutions offered by drone imagery is one of the main factors contributing to its popularity, particularly for agricultural applications.
Unfortunately, acquiring images at low flying heights has a few drawbacks. First, multiple images are required to cover a large area (e.g. an orchard in the case of drones or a city in the case of conventional aerial surveys). The second drawback (which is related to the first) is that the images are acquired over a period of time (e.g. a few hours or even multiple days). Invariably the conditions (e.g. sun illumination and angle) under which the images are acquired change during the acquisition period, which introduces inconsistencies among the images. Figure 1 shows a case where aerial photographs covering Cape Town were acquired over several hours. Even at this small mapping scale (i.e. zoomed out view) one can clearly see how the illumination changed during the aerial survey.
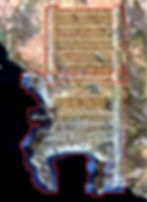
The same thing happens in drone surveys, albeit to a less noticeable degree thanks to the shorter flying times. Image processing software is usually used to mosaic the individual images and to cosmetically reduce inconsistencies among images by applying "colour matching" and other dodgy techniques (one technique is actually called "dodging"). This prepares the imagery for visual interpretation, but dramatically alters the pixel values (brightness and colour characteristics) of the images.
Given that image analyses rely on quantitative comparisons of pixel values to "measure" the characteristics of target objects (e.g. the growth vigour of trees in different parts of an orchard), it make little sense to compare two images acquired at different times, and under very different conditions, with one another. Or to use imagery that has undergone cosmetic modifications.
A third challenge with dealing with multiple images is that each object on the ground is viewed from at least two different angles (due to the overlap among images). This causes a number of distortions when the images are mosaicked. Compared to aerial surveys, this problem is usually more pronounced in drone imagery as a larger degree of overlap and wider angled lenses are typically used. This type of distortion is great if you are a cubist painter -- see Picasso’s portrait of a woman below, representing views from different perspectives. For obvious reasons, viewing things from different and inconsistent angles is not ideal for quantitative analyses!
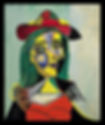
To address these issues, Dugal Harris (one of my gifted PhD students) recently developed a technology to remove most of the inconsistencies in aerial imagery so that it can be used for quantitative analyses. Details of this ground-breaking technology can be found in a recent paper published in the International Journal of Remote Sensing. In essence each aerial image is calibrated to a near-concurrent satellite image representing surface reflectance. The satellite image is normally of a much (e.g. 1000 times) lower resolution than the aerial image. For instance, to calibrate 50cm historical aerial imagery, Dugal used 500m resolution MODIS imagery as it is radiometrically very well calibrated/corrected, acquired on a daily basis and freely available.
Below (Figure 2) is a zoomed-in version of Figure 1. Clicking on the right arrow on the image will show the effect of the calibration. The MODIS image used for calibration is shown outside of the red lines (notice the much lower resolution).
Below is a before-and-after video of a more detailed area. Notice how the seam lines (sharp transitions between images) and the effect of the wide angle lenses (light to dark gradient from image centre of to edges) vanishes.
The technique works extremely well, much better than we expected! Even better results can be obtained using freely-available surface reflectance imagery provided by the Landsat-8 and Sentinel-2 constellations. Sentinel-2 imagery, at 10 m resolution, is ideal for calibrating ~5 cm resolution drone imagery. Watch this space for updates on this exciting new technology.
In the meantime, be cautious of the fact that aerial and drone imagery are generally not well calibrated. Any outputs of quantitative analyses (e.g. vegetation indices) of such imagery should be interpreted with great care, especially when comparing images that were acquired at different times of the day, or year.
This article was originally published on www.remotesensing.blog, which is devoted to articles about the use of remote sensing and other geospatial technologies for agricultural and related applications.
See related posts: